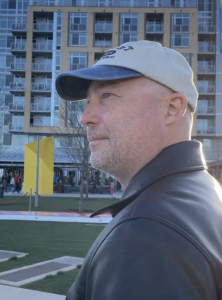
Alexander Mitrophanov
In the September 2022 Bulletin issue, we introduced a new “Invitation to Research” section, kicked off by Alexander Y. Mitrophanov, Senior Statistician at the Frederick National Laboratory for Cancer Research, National Institutes of Health, USA. Alex invited members to collaborate on Quantitative Perturbation Theory for Stochastic Processes, which since created “very meaningful” follow-up. Alex now writes another Invitation to Research:
Statistics, Stochastics, and Data Science for Systems Biology
It has been quite a while since systems biology stopped being a buzzword and became an established research field (as illustrated, e.g., by the fact of existence of a Systems Biology Department at Harvard). While systems biology is a “mindset rather than a tool set” (paraphrasing one of the field’s leaders, Yoram Vodovotz), its success depends on the tools it uses. And the needed mathematical and statistical tools may not always be immediately available. This article reflects my personal perspective based on years of experience working in that broad field.
In systems biology, there are two main methodological approaches: bottom-up and top-down. Due to my familiarity with the former, I will mainly focus on it here. “Bottom-up” means that we start with a (known or assumed) molecular or cellular mechanism underlying some biological system, build a mathematical model, fit it to the available experimental data, and then attempt to make predictions or draw conclusions about the behavior of the system as a whole. The computational predictions can, and should, be then tested experimentally, and the cycle of model improvement, prediction, and testing can continue as needed. In “classic” systems biology, the mathematical model often is a system of nonlinear ordinary differential equations (ODEs), so fitting it to the data is an exercise in nonlinear regression (more precisely, in inference for dynamical systems). And here, right away, we run into questions. For example, how many data points per one model parameter do we need to have? Will the rule of thumb of 10 data points per parameter apply here? Recently, a remarkable work challenged this rule of thumb for logistic regression [1], illustrating the depth of this question. This is related to the general question of sample size and power analysis for nonlinear models, and rigorous answers to it are actively researched. Now, if we obtained some estimates for our nonlinear model’s parameters, what is the best way to compute confidence and prediction intervals for the model’s outputs? Bootstrapping is often an option, but how can we derive accurate enough, and general enough, analytic approximations to reduce the computational burden? It should be noted that, in systems biology, model fitting is not always straightforward sum-of-squares minimization, but sometimes can be “fitting with a twist,” where the “twist” depends on the problem being solved. For example, in one study, we needed to keep adjusting the ODE system’s initial conditions during fitting to make sure that the initial conditions always correspond to the system’s steady state [2]. In another study, the initial condition was not an issue, but the possibility of overfitting was, so we implemented a neural-network-style stopping criterion in our ODE-model fitting algorithm [3]. It would be good to know how the properties of the resulting fits (such as consistency) depend on the “twist” being used. If we go beyond ODEs and enter the world of partial differential equations, all these problems only become harder.
A major extension of the ODE-based approach is stochastic modeling, where we use a random process to simulate system kinetics. (The ODE here can be regarded as an approximation when the number of system components—molecules or cells—is very large.) Often, stochastic models in systems biology are inspired by the chemical master equation formalism and thus constitute a continuous-time Markov chain. On the theory side, this area provides rich opportunities for studying different kinds of approximations and limits. Notably, such results may also apply outside systems biology (e.g., in population dynamics). On the more practical side, current research focuses on algorithms and computational strategies to simulate stochastic biomolecular systems in efficient ways, which becomes an issue for large systems (e.g., [4]). And, of course, there’s the ever-present question: how do we fit stochastic models to experimental data, and what are the statistical properties of the resulting fits? Being an active area of research (e.g., [5]), this topic can benefit from new and improved solutions.
Finally, in top-down systems biology, we start with data sets and try to elucidate robust data patterns, understand the dependencies between variables, and even infer the structure of the underlying biological system. This is probably the most mature area of application of statistical methods in systems biology. Successes here have been numerous, but new challenges continue to emerge. Due to improvements in experimental methodology, biological data keep growing in both amount and complexity, and new research questions arise. This necessitates new developments not only on the side of analysis methodology, but also on the side of data storage, data security, data sharing, and computational resources. This is where what we call statistics meets what many call data science. One recent example is the problem of integration of multimodal biomedical data (e.g., data from genomics studies, physiological measurements, medical imaging, and electronic patient records).
I firmly believe that statistical science and systems biology will continue to enrich and strengthen each other for years to come.
If you are interested in this area and would like to work together on these ideas, get in touch: alex.mitrophanov@nih.gov. If you have an Invitation to Research of your own, email bulletin@imstat.org.
References
1 Sur P., Candès E. J. (2019) A modern maximum-likelihood theory for high-dimensional logistic regression. Proc Natl Acad Sci 116: 14516–14525.
2 Kato A., Mitrophanov A. Y., Groisman E. A. (2007) A connector of two-component regulatory systems promotes signal amplification and persistence of expression. Proc Natl Acad Sci 104: 12063–12068.
3 Mitrophanov A. Y., Szlam F., Sniecinski R. M., Levy J. H., Reifman J. (2016) A step toward balance: thrombin generation improvement via procoagulant factor and antithrombin supplementation. Anesth Analg 123: 535–546.
4 Anderson D. F., Ehlert K. W. (2022) Conditional Monte Carlo for reaction networks. SIAM J Sci Comput 44: A993–A1019.
5 Vo H. D., Fox Z., Baetica A., Munsky B. (2019) Bayesian estimation for stochastic gene expression using multifidelity models. J Phys Chem B 123: 2217–2234.