Xiao-Li Meng chats some more about ChatGPT, following his XL-Files in the April/May 2023 issue:
Since my first encounter with it in March, ChatGPT, along with its many emerging siblings and cousins (some out of wedlock), has rapidly evolved into a “promptbot,” by which I’m not referring only to the way chatbots have given rise to the concept of “prompt engineering”. More broadly, they have prompted a whole spectrum of actions, from the outright banning and panning of their uses to intensely exploring and exploiting their potential. History has shown that humanity has rarely (ever?) resisted the allure of our technological achievements. Banning technology is about as effective as telling a teenager not to watch an R-rated movie. The only way to suppress a technology that has already piqued the public interest is to introduce a better one: rather than fearing it, steering it in the right direction, first studying its boons and dooms.
I therefore was delighted when my fearless and tireless colleague, Lucas Janson, initiated a summer reading group on studying the impact of generative AI on the field and practice of statistics. Of course, a summer is too short to study everything, and hence after some venting and voting, we settled on the following weekly schedule:
7 & 14 June Overview: Model principles of generative AI
21 June Overview: What’s out there?
28 June Overview: Prompt engineering
5 July Assistive tool: Writing code
12 July Assistive tool: Writing text
19 July Assistive tool: Making visualizations
26 July Speculative tool: Searching academic literature
2 August Assistive tool: Cautions/pitfalls
9 August (No meeting: JSM)
16 August Research topics: Model interpretation
23 August Research topics: Calibrating uncertainty
Except for the first two sessions, which were led by a faculty member and two students, each topic was covered by a team of two students, or by a student–faculty pair. I joined the one on “What’s out there?”, partly because of my access to the diverse editorial board of Harvard Data Science Review (HDSR), which is in the midst of organizing a special issue on “Future Shock: Grappling With the Generative AI Revolution.” (This is the first open call from HDSR, so please don’t miss the opportunity to submit: https://hdsr.mitpress.mit.edu/). Thanks to the superb human intelligence provided by PhD student Ritwik Bhaduri, we ended up with nearly 50 dense slides, covering eight functionalities, as summarized in the opening slide:
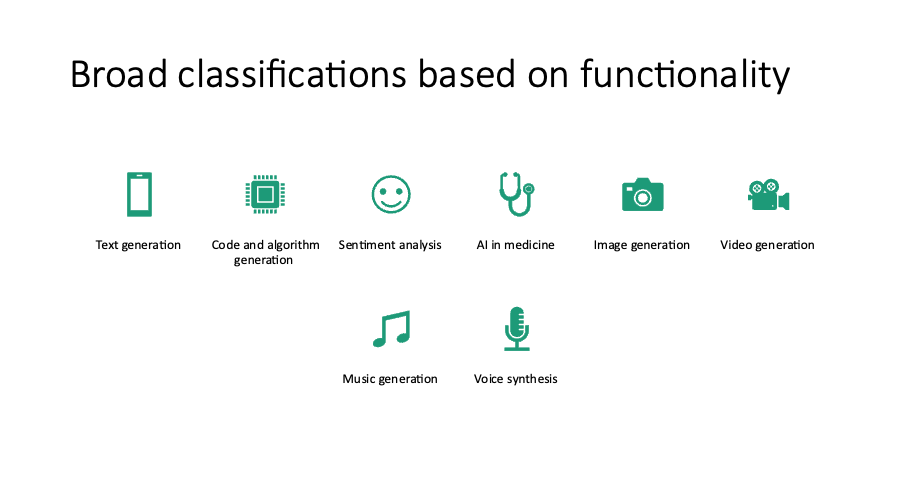
Eight functionalities of AI: text generation; code and algorithm generation; sentiment analysis; AI in medicine; image generation; music generation; and voice synthesis
We began with text and code generation because they are essential tools for those diligently working toward their academic degrees or tenure, as well as for those of us who have not found a lifestyle more satisfying than generating texts with strategically embedded Greek letters. The versatility of generative AI shines through its wide range of functions and adaptability within each function. For instance, with just text generation, GPT-4 can create, capsulize, condense, compare, contrast, and critique content. And as technological advancements continue, we can anticipate even more capabilities, especially with a plethora of plugins regularly emerging.
We highlighted the sentiment analysis to show how generative AI can be employed to produce research data before generating text or code. We cited a study on how GPT-4 can classify the sentiment of news headlines—positive, negative, or neutral—to predict stock market trends for the following day. Predictions are inherently risky. However, it’s a safe bet that for sentiment analysis, generative AI will surpass and replace many human “classifiers”. Envisioning GPT-n as a superlative “wisdom of the crowd” would not be a hallucination, especially as n grows. After all, GPT-n is trained on a vast amount of data that surpasses what any group of humans can handle (without the help of generative AI). If our collective sentiments sway the stock market, it’s more logical to trust a massive synthesizer like ChatGPT over individual human judgment. This isn’t to claim that AI surpasses human intelligence. It’s merely a nod to two facets of human intelligence: the collective wisdom of humanity and the unique intellect of individuals. ChatGPT essentially grants individuals unprecedented access to a digitized form—however incomplete or biased—of our collective knowledge, available nearly anytime and anywhere.
There’s a well-known Chinese saying, “힛몸높튄슁,땅몸瀨모좋”. While a pun-preserving translation is virtually impossible, GPT-4 offers a poetic rendition: “In unity of three craftsmen’s thought, a sage’s wisdom is forth brought.” I’m fond of the term “unity,” as it encapsulates generative AI’s cohesive wisdom synergy, contrasting human attempts at consensus. How often do we see a group of experts—say 30 (much less 30 million)—come to a unanimous decision quickly, or at all? This synthesized intelligence, while efficient, may at times lack the depth of diverse human perspectives. But the value of swift consensus can’t be understated, especially when human biases, self-interests, or egos obstruct decision-making. Even in an ideal world driven solely by the greater good, promptly gathering and synergizing insights from a large group of experts remains a dream (or nightmare).
The time-saving advantage of using ChatGPT is undeniable, especially for those intrigued by countless challenges in data science. While churning out more articles shouldn’t be the sole aim of (academic) research, very few institutions would grant tenure based on a small number of high-quality publications. It’s thus a reasonable prediction that the volume of academic articles will surge alongside the progression of GPT-n.
Is the overall quality of research articles also on an upward trajectory? That’s a vastly more challenging hypothesis to test, especially since assessing quality necessitates its own set of comprehensive research. One would hope that as quantity increases, quality doesn’t proportionately decrease. But that might remain merely a hope.
Consider the need to search for and summarize literature in preparing research articles, a task for which ChatGPT is known to hallucinate at times. To see how much progress GPT-4 has made over GPT-3.5, I prompted both with the same request: “Provide some representative articles by Xiao-Li Meng” (on Aug 20, 2023). GPT 3.5 came back with a list of five. The first four were accurate, but the last one,
“Gelfand, A. E., & Meng, X.-L. (1990). Model choice in generalised linear models via noninformative priors. Biometrika, 77(2), 249–261”
…is a complete fabrication. I never had the opportunity to co-author with Alan Gelfand. The article title doesn’t exist (nor would it be adopted by any reputable statistician). Furthermore, the page range provided in 1990’s Biometrika straddles two completely unrelated articles.
Nevertheless, compared to a similar test I conducted in March, GPT-3.5 has shown notable progress. Back then, all four articles it provided were fictional. Worse still, the four titles appeared plausible or even credible, which is perilous since apparent authenticity demands discernment to identify false references. Although verifying a reference’s legitimacy is typically straightforward (for those who at least glance at what they cite), when ChatGPT is employed to explore unfamiliar territories, the risk to research quality magnifies. Fictitious information, if unchecked, can become fodder for future AI training, perpetuating inaccuracies, and ultimately, like repeated lies, creating an illusion of truth.
While GPT-3.5’s performance in August outshone its earlier version, the optimism was short-lived. GPT-4 responded to the same prompt with six articles, mis-attributing four of them. Two of these errors amusingly reassigned my co-authors, with a Harvard classmate (Andrew Gelman) replaced with a Chicago colleague (Wing Wong), and a Chicago advisee (David van Dyk) with my Harvard adviser (Donald Rubin). Another amusing misrepresentation,
“Multiple Imputation in Practice: Comparison of Software Packages for Regression Models With Missing Values. Meng, X.-L., Rubin, D. B. (1992). The American Statistician, 45(3), 186–202”
…is a genuine title from The American Statistician but was published two decades later by Horton and Lipsitz (2012, Vol 55, 244–254). Although my PhD advisor Don Rubin and I are known to be “multiple imputers” (thankfully Don didn’t venture into “serial imputation”), the term “software” appeared once in Don’s extensive list of article titles, and not at all in my publications. This fact should significantly diminish the likelihood that either of us would publish an article on “comparison of software packages.” However, when an AI learns patterns primarily through exhaustive training, its ability to discern what shouldn’t be there—such as the absence of certain topics in our publications—depends on its training on what is missing, a negation learning that seems to be beyond GPT-4’s current capabilities.
In general, GPT-4 is widely recognized as a leap forward from GPT-3.5. In this context, however, the term “forward” would be apt only if I were tempted to pad my list of publications through “multiple imputations.” Of course, such a notion should not even cross anyone’s mind. However, my playful experiment is not without a serious message. As tools like ChatGPT become more pervasive, the risk of erroneous outcomes, whether unintentional or malicious, is likely to accelerate, notwithstanding technological advancements. While this isn’t cause for panic, it should compel us to approach information with heightened scrutiny, filtering it through our critical thinking and discernment. If a significant number of human beings make this a habit, our collective wisdom as a species might just grow. Such progress would truly be a testament to the potential of humanity—after all, the primary goal of AI is not to supplant, but to amplify human intelligence on both collective and individual scales.
Lastly, lest anyone assumes I’ve been infected by ChatGPT’s penchant for hallucinations, let me share the ChatGPT-3.5 poem I promised in my April/May XL-Files. It serves as a reminder that our profession could benefit from a dose of GPT’s imaginative flair, especially the kind that nudges us out of our usual boundaries:
“All data has [sic] stories, some mistold
Messy facts, some can unfold
All models simplify, some more sound
Fallible assumptions, some more profound
Methods serve, some versatile
Interpretations, some contrived, some worthwhile”