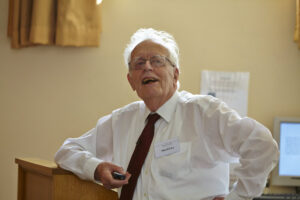
Sir David Cox in 2014 at his 90th Birthday Symposium. Photo courtesy of Nuffield College Oxford.
Sir David Roxbee Cox died suddenly at his home in Oxford on January 18, 2022, leaving Joyce, his patient and supportive wife of almost 75 years, and their four children. David was so engaged and energetic in research that his death came as a shock.
David was born on July 15, 1924, in Birmingham, England, and held academic positions at University of Cambridge, Birkbeck College London, Imperial College London, and Nuffield College Oxford. He spent 15 months in the United States in 1955–56, visiting the University of North Carolina, Princeton, and UC Berkeley. During that time, he presented a Special Invited Lecture to the IMS, which became his highly influential 1958 paper, “Some problems connected with statistical inference” (46)1 [Numbered references refer to David’s publication list at Nuffield College1.]
His seemingly boundless energy and enthusiasm, his brilliant mind, and a great deal of hard work, gave the world a remarkable oeuvre of contributions to all areas of statistical science and many of its applications. It is impossible in a short piece to do justice to these, and more detailed accounts will no doubt appear in other venues. A short article in the previous issue2 highlights his key awards, although special note must be taken of the Royal Society’s Copley Medal (2010), its highest honour. Previous winners include Gauss, Fisher, Bohr, and Einstein. Here we sketch some of his contributions to stochastic processes, foundations of inference, and statistical theory.
The doubly-stochastic Poisson process, also known as the Cox process, appeared in a remarkable paper in 1955 read to the Royal Statistical Society (36). The paper also includes a precursor to generalized linear models, the use (without comment) of the confidence distribution, attention to graphical displays, variance components, model fitting, model assessment, and so much more. In the discussion, Pearson commented, “it might have been a better policy to have narrowed his field of discussion and provided more illustrative material”, but other comments were more astute — those of Bartlett on doubly stochastic processes are especially illuminating. David’s grounding in stochastic processes suffused and influenced his work throughout his life. His insightful analysis of dependence in large data sets (361) is discussed by modelling the accumulation of data, and its variability, as a stochastic process with potentially long-range dependence. In his Statistical Science interview3 he noted that his work on the proportional hazards model was directly informed by his background in stochastic processes.
David’s work on inference brought clarity to a subject whose foundations were fragmented, sometimes incomprehensible, and occasionally badly flawed. Such was his modesty that he attributed many of the key ideas in his more philosophical papers and books on statistical inference to Fisher. The abstract of his masterfully lucid 1958 paper (46) notes: “It consists of some general comments, few of them new, about statistical inference. Parts of the paper are controversial; these are not put forward in any dogmatic spirit.” In spite of these disclaimers, the paper is a landmark in the development of the foundations of inference. It covers many aspects of current relevance, including formal discussion of confidence distributions, but is best known for its convincing demonstration of the need for appropriate conditioning in order to ensure scientifically relevant conclusions from statistical inference. This led directly to a long and important philosophical discussion, initiated by Birnbaum, on the role of the likelihood principle in inference and the interplay between frequentist and Bayesian inference. The paper also revealed that conditional inference is usually incompatible with ideas of optimality that remain popular today. The question of where to limit the conditioning is a challenging one, discussed for example in (226, §2.4). In the simplest setting, an arbitrarily granular choice renders each individual uninformative about others, while too coarse a conditioning typically yields conclusions irrelevant to the question at hand. When there are many nuisance parameters the appropriate conditional formulation becomes particularly elusive, although the conceptual argument for distinguishing samples of varying degrees of information remains compelling. A first attempt appeared in (46) and subsequent work sought to achieve the appropriate conditioning approximately.
In (139) he used an approximating curved exponential family to derive what he called a local ancillary statistic, and obtained an approximation to the distribution of the maximum likelihood estimator, conditional on this statistic. Several other papers in the same issue of Biometrika tackled related problems, and the so-called p*-approximation emerged as a common thread. David’s interest in this was not focussed on the impressive numerical accuracy of the higher-order approximations, but on the implications of their structure for the foundations of inference. He refused to be dazzled by intricate mathematics or clever computation, unless it was demonstrably effective for solving what he might call “real problems”. His pair of books with Barndorff-Nielsen (188, 226) contain a great deal of challenging mathematical detail, but are also full of statistical insight and enlightening examples.
David said that none of his books were written to be textbooks, although the very influential Theoretical Statistics (113) with David Hinkley is an exception. The emphasis on concepts of statistical inference and their relevance for applications, along with the parallel de-emphasis on mathematical details, distinguishes it from most books on statistical inference or mathematical statistics. It places likelihood and sufficiency at the centre of the theory of statistics, and may be the first text to clarify the distinction between significance testing, as developed by Fisher, and Neyman and Pearson’s approach to hypothesis testing, treating both in considerable detail. Every potential principle of statistical inference is first explained, and then challenged, so effectively that the book can seem a collection of counter-examples. This is consistent with David’s firm belief that the foundations and methods of statistical inference must be continually challenged and evaluated against their utility for applications, a point made strongly in his 2006 book (315), and again in (363). His writing on statistical significance and p-values seemed to need repeating for each new generation; a modern and concise account was published in 2020 (378).
David’s contributions flowed smoothly between foundations of inference, theoretical analysis, development of methodology, and applications. He himself did not view these aspects as separate. A prominent example of this coalescence of ideas was his development of logistic regression (48, 49, 98), a topic so ingrained in modern statistical training that the ingenuity in its conception can be easily overlooked. A key aspect in the development was to specify sufficient statistics for the regression coefficients that coincide with those of a normal-theory linear model. The logistic construction emerges as the unique model for binary data that produces such unification, and an elegant theory of conditional inference then ensues, evading maximum likelihood fitting. This work is just one of his many unifying accomplishments; it reduces in the simplest special case to Fisher’s (1935)4 conditional analysis of the 2×2 contingency table, and leads in (90, 98) to the observation that all exponential-family responses can be treated in essentially the same way. A more flexible version, allowing one to renounce these simple sufficient statistics, was proposed by Nelder and Wedderburn (1972)5 in the form of generalized linear models.
David was best-known for his 1972 paper (106) on the proportional hazards model. Its impact was both fundamental and immediate. It is ranked 16th in Nature’s list of most cited papers of all time in all fields, and was cited in the awards of the inaugural International Prize in Statistics (2016), the BBVA Foundation Frontiers of Knowledge Award (2017) and the Kettering Award (1990) for the most outstanding recent contribution to the diagnosis or treatment of cancer. As was characteristic of all his work, the elegant ease with which the results seemed to materialise partially masked the fundamental leaps involved in their inception, and the remarkable command of intuition, insight and technique that brought them to fruition. Once again, the motivating applications were the basis for foundational development, and partial likelihood emerged in 1975, encompassing conditional6 and marginal7 likelihood analyses.
His work remained current, and sometimes ahead of its time, hindered by prevailing computational considerations. In 1975 he gave an early elucidation of post-selection inference (115), demonstrating serious loss of inferential guarantees that sometimes arise when the research question to be studied is selected in the light of the data, and establishing the theoretical properties of sample-splitting in the simplest example. A key idea presented in passing in (90), further elaborated in (114, 193), resurfaced when he gave a totally new perspective on the sparse high-dimensional regression problems routinely encountered in genomics research. If multiple low-dimensional models are compatible with the data, his view was that one should aim to report them all, rather than a single model effective for prediction. This underpins the development of confidence sets of models in (371, 374).
David’s influence on science and statistical science was extraordinary, and his work will repay careful study for many years to come. His death leaves science much the poorer, without his keen judgment and unfailing curiosity; without his capacity to set the course of advancing knowledge with a single decisive contribution. Those fortunate enough to have crossed his path, professors and students alike, will remember a modest gentleman, keenly interested in everything scientific, thoughtful and perhaps a little bit shy. Until he stood up to deliver his talk. Then, one had a glimpse of his formidable intellectual energy and creativity, and remembered that talk for a very long time.
We miss him.
—
Written by Heather Battey, Imperial College London, and Nancy Reid, University of Toronto
References
1 Numbered references refer to the publication list at Nuffield College: https://www.nuff.ox.ac.uk/Users/Cox/Publications.html
2 IMS Bulletin (2022), 51, 2
3 Reid, N. (1994). Statist. Sci., 9, 439–455.
4 Fisher, R.A. (1935). J. R. Statist. Soc., 98, pp. 39–54.
5 Nelder, J. and Wedderburn, R. (1972). J. R. Statist. Soc. A, 135, pp. 370–384.
6 Bartlett, M. S. (1936). Proc. Roy. Soc. A, 154, pp. 124–137.
7 Fraser, M. S. (1968). The Structure of Inference. John Wiley & Sons.