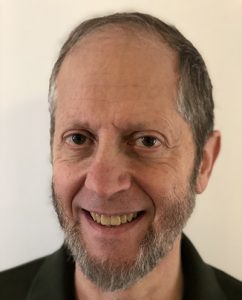
Paul Rosenbaum
Paul R. Rosenbaum is the Robert G. Putzel Professor in the Department of Statistics at the Wharton School of the University of Pennsylvania, where he has worked since 1986. Prior to this, he worked at the US Environmental Protection Agency, the University of Wisconsin–Madison, and the Educational Testing Service. He is the author of three books: Observational Studies (Springer, 2nd edition, 2002), Design of Observational Studies (Springer, 2010), and Observation and Experiment: An Introduction to Causal Inference (Harvard University Press, 2017). Paul received his PhD in Statistics from Harvard University in 1980, with Donald B. Rubin and Arthur Dempster as thesis advisors. He delivered the Fisher Lecture and received the R. A. Fisher Award in 2019, and the George W. Snedecor Award in 2003, both from COPSS.
Paul’s Medallion Lecture will be given at the Joint Statistical Meetings in Philadelphia in August.
Replication and Evidence Factors in Observational Studies
Observational studies are often biased by the failure to adjust for a covariate that was not measured. A series of studies may replicate an association because the bias that produced this association has been replicated, not because a treatment effect has been demonstrated. If a limited sample size is not the major problem in an observational study, then an increase in sample size is not the solution. To be of value, a replication should remove, or reduce, or at least vary a potential source of bias that resulted in uncertainty in earlier studies.
Having defined the goal of replication in this way, we may ask: Can one observational study replicate itself? Can it provide two statistically independent tests of one hypothesis about treatment effects such that the two tests are susceptible to different unobserved biases? Can the sensitivity analyses for these two tests be combined using meta-analytic techniques as if they came from unrelated studies, despite using the same data twice? Can such a combination provide stronger evidence that an association is an effect caused by the treatment, not a bias in who was selected for treatment? When this is possible, the study is said to possess two evidence factors. A study has two evidence factors if it permits two (essentially) statistically independent analyses using the same data that are affected by different types of unmeasured biases. More specifically, the sensitivity analyses for the two factors must be capable of combination as if they came from different unrelated studies, despite using the data twice. This latter condition is in some ways stronger than statistical independence, in other ways weaker.
The talk is divided into three parts:
(i) a brief, largely conceptual discussion of replication in observational studies;
(ii) a longer, more technical discussion with results about and practical examples of evidence factors,
(iii) consideration of algorithmic aspects of building study designs with evidence factors.
Some References about Replication and Evidence Factors
Rosenbaum, P. R. (2001). Replicating effects and biases. American Statistician, 55(3), 223–227.
Rosenbaum, P. R. (2010). Evidence factors in observational studies. Biometrika, 97(2), 333–345.
Rosenbaum, P. R. (2011). Some approximate evidence factors in observational studies. Journal of the American Statistical Association, 106(493),285–295.
Zhang, K., Small, D. S., Lorch, S., Srinivas, S., Rosenbaum, P. R. (2011). Using split samples and evidence factors in an observational study of neonatal outcomes. Journal of the American Statistical Association, 106(494), 511–524.
Zubizarreta, J. R., Neuman, M., Silber, J. H., Rosenbaum, P. R. (2012). Contrasting evidence within and between institutions that provide treatment in an observational study of alternate forms of anesthesia. Journal of the American Statistical Association, 107(499), 901–915.
Rosenbaum, P. R. (2015). How to see more in observational studies: Some new quasi-experimental devices. Annual Review of Statistics and Its Application, 2, 21–48.
Rosenbaum, P. R. (2017). The general structure of evidence factors in observational studies. Statistical Science, 32(4), 514–530.
Karmakar, B., Small, D. S., Rosenbaum, P. R. (2019). Using approximation algorithms to build evidence factors and related designs for observational studies. Journal of Computational and Graphical Statistics, 28(3), 698–709.
Karmakar, B., Small, D. S., Rosenbaum, P. R. (2020). Using evidence factors to clarify exposure biomarkers. American Journal of Epidemiology, to appear.