Darryl D. Holm is a Professor of Applied Mathematics at Imperial College London. He writes this YoungStatS contribution, giving an overview of how transport noise is used in modeling fluid dynamics:
In this contribution, I will give an overview of some old and new approaches related to the use of transport noise in the stochastic modelling of fluid dynamics. Recent advances on this topic were presented by four young scholars at the One World YoungStatS webinar, supported by FENStatS, the Bernoulli Society and the Institute of Mathematical Statistics, in November 2023 (see https://youngstats.github.io/post/2023/10/14/stochastic-fluid-dynamics/).
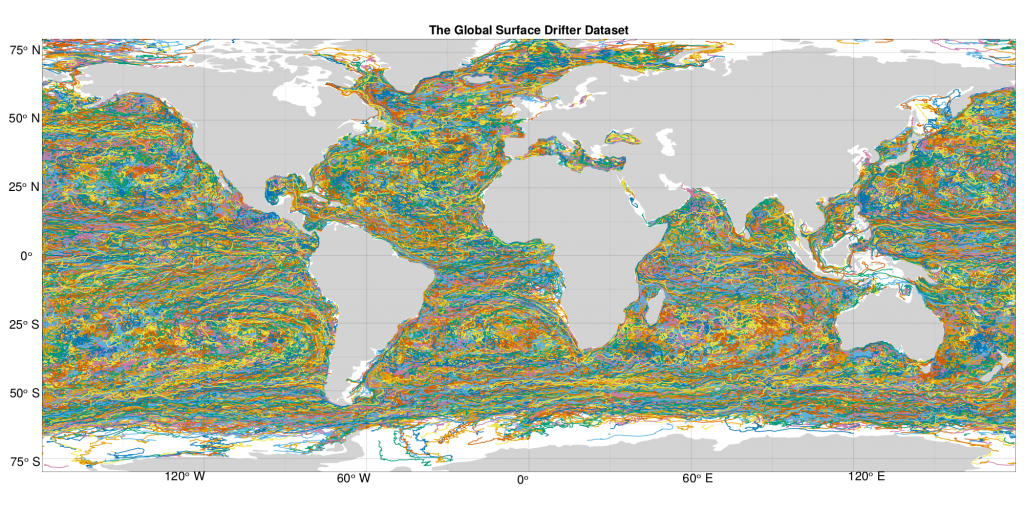
Trajectories from the National Oceanic and Atmospheric Administration Global Drifter Program are shown, in which
each color corresponds to a different drifter. Image courtesy of NOAA’s Global Drifter Program.
Transport noise in fluid dynamics has several forebears in turbulence theory, as in the early 1990s, Kraichnan and Pope sought to explain the anomalous spectral scaling in turbulent flows by computationally simulating stochastic transport of passive scalars and stochastic Lagrangian particle paths. At about the same time, Brzeźniak, Capiński and Flandoli introduced stochasticity into active transport of vorticity in the two-dimensional (2D) Navier–Stokes equation. Cipriano and Cruzeiro established a stochastic variational principle for the 2D Navier–Stokes equation and Constantin and Iyer derived a probabilistic representation of the deterministic three-dimensional (3D) Navier–Stokes equations based on stochastic Lagrangian paths. Stochasticity eventually found its way into both the motion and transport equations for 3D fluid dynamics via Hamilton’s variational principle in Holm (2015). The author named his variational stochastic model for fluid transport as Stochastic Advection by Lie Transport (SALT).
In the works of several authors, stochasticity of finite dimensional system of differential equations was soon made Hamiltonian and stochastic Hamiltonian dynamical systems were reformulated in the Lie–Poisson framework. It was natural to expect that stochastic Hamiltonian dynamical systems should also emerge from a phase-space version of Hamilton’s principle. A variant of such a theorem was proved in Lázaro-Camí and Ortega, and another one for Lagrangian systems by Bou-Rabee and Owhadi. These discoveries quickly led Cruzeiro, Holm and Ratiu to derive the entire spectrum of finite-dimensional geometric mechanics, from reduction by symmetry of the Lagrangian to derive a stochastic version of the Euler–Poincaré variational principle.
Following the recognition of Lie-group reduction of Hamilton principles for deriving finite-dimensional stochastic Euler–Poincaré (EP) variational principles, it was natural to attempt the same for the Eulerian representation of fluid dynamics, which was soon performed separately by Holm, and Chen, Cruzeiro and Ratiu. As it turned out, in 2D, these variational approaches recovered the planar stochastic Euler vorticity equations with transport noise studied earlier.
When all is said and done in following the EP approach, the stochasticity appears only in the transport velocity of the material loop in Kelvin’s theorem for the conservation of circulation around material loops in 3D Euler fluid dynamics. Thus, the EP variational approach is a natural one for deriving stochastic fluid dynamics equations with transport noise.
The next natural step was for Crisan, Flandoli and Holm to examine the analytical properties of the newly derived EP Euler fluid equations with transport noise in three dimensions. Remarkably, because the noise involved Lie derivatives, the analytical properties of the stochastic 3D Euler equations with SALT turned out to be essentially the same as for the deterministic case. This agreement in analytic regularity was not entirely a surprise, though, as the SALT model for the Euler equations had been earlier derived by applying the mathematical method of homogenisation.
The recent work of Crisan, Holm, Leahy and Nilsen (2022a) aims to transfer the fundamental properties of deterministic fluid dynamics derived by Hamilton’s principle into a formulation on geometric rough paths. Another work by the same authors (2022b) analyses the solution properties of Euler fluid dynamics on rough paths and demonstrates the efficacy of this approach by producing previously unavailable results.
Recent work on stochastic Geophysical Fluid Dynamics has led to collaboration to investigate Stochastic Dynamical Data Assimilation, for quantifying and reducing uncertainty in numerical simulations of weather, climate and ocean circulation. Remarkably, this has also been found to work well for quantifying and reducing uncertainty in applications of stochastic shape analysis for image registration. In particular, foundational development of the new science of Stochastic Geometric Mechanics for spatial smooth invertible maps with stochastic time dependence has produced fruitful applications to uncertainty quantification and reduction of uncertainty via data assimilation in fluid dynamics.
A promising goal in the future is to apply stochastic geometric mechanics for the derivation, analysis, numerical simulation and assimilation of computational data, such as satellite observations of various types of transport like heat, mass, colour, texture, and other observable order parameters in upper ocean dynamics. Reaching this goal will require a variety of developments in modelling and data assimilation, related to transport noise in fluid dynamics.
Transport noise has now found important applications in oceanography under the auspices of the European Research Council Synergy Grant, entitled “Stochastic Transport in Upper Ocean Dynamics” (STUOD), see, e.g., https://www.imperial.ac.uk/ocean-dynamics-synergy/
Main references:
Crisan, D., Holm, D.D., Leahy, J.M. and Nilssen, T., 2022. Variational principles for fluid dynamics on rough paths. Advances in Mathematics, 404, p.108409. https://doi.org/10.1016/j.aim.2022.108409.
Crisan, D., Holm, D.D., Leahy, J.M. and Nilssen, T., 2022. Solution properties of the incompressible Euler system with rough path advection. Journal of Functional Analysis, 283(9), p.109632. https://doi.org/10.1016/j.jfa.2022.109632.
Holm, D.D., 2015. Variational principles for stochastic fluid dynamics. Proc. Roy. Soc. London A, 471(2176). http://doi.org/10.1098/rspa.2014.0963.