Layla Parast writes:
Fall is the time for apples, pumpkin spice, crunching leaves, and—for me—freshmen. Some people strongly dislike teaching freshmen, but I love it. I feel like a summer camp counselor again, except that this time I don’t have to worry about kids being pushed in pools or whacked in the head with a tennis racket. Like a mother hen, I extend my wing to shield all of my baby chicks, and teach them how to find food and escape predators. Yes, you do need to eat breakfast. No, you cannot survive on two hours of sleep every night. Yes, you should actually read the syllabus. No, please don’t eat that brownie you found on the windowsill.
The freshmen I teach are Statistics and Data Science majors. They choose to take my course, Introduction to Data Science, and they are bursting with excitement to learn. I co-created this course with a colleague, Sally Ragsdale, years ago, at my department chair’s request: she wanted us to design a course to introduce students to “data science” without teaching them any statistics, since the statistics curriculum begins during the second semester. After hearing this request from my chair, I returned to my office and promptly Googled: “What is data science?” (true story!).
First of all, I teach the students to code in R (they also learn Python in a different course). They learn to visualize and describe data, create R markdown reports, work with tibbles, dplyr, relational data (merging datasets), date/time formatting, reshaping data, string manipulation, for loops, functions, and simulations. At the end of the course, the students build and publish their own R Shiny app.
The course also focuses on the role of data science in our society, and on the ways in which our past experiences and perspectives can affect how we collect and analyze data, often unconsciously. In one of my favorite lectures, we read and discuss an excerpt on communicating context from Chapter 6 of the book Data Feminism by Catherine D’Ignazio and Lauren F. Klein (https://data-feminism.mitpress.mit.edu). This excerpt displays a barplot showing rates of mental health diagnoses by race among people incarcerated for the first time in NYC jails between 2011 and 2013. The figure is displayed twice, with two different titles: one title is “Mental Health in Jail: Rate of mental health diagnosis of inmates”, and the other title is “Racism in Jail: People of color less likely to get mental health diagnosis”. D’Ignazio and Klein argue that in the first title, the use of the word “inmates” is dehumanizing and fails to communicate the study results. They argue that it is our “responsibility to connect the research question to the results and to the audience’s interpretation of the results.”
Full disclosure: this perspective makes me uncomfortable. For over a decade, I worked at the RAND Corporation, an organization whose tagline emphasizes “Objective analysis.” My gut instinct is to prefer the first title, since it states the purpose of the study in a (seemingly) objective way, rather than the researchers’ conclusions about the study (which could be perceived, rightly or not, as subjective). But is my discomfort with the second title really rooted in my past work experience, or is there something deeper at play? It’s important for me to emphasize—both in this column, and to my students—that there is no right or wrong title. The primary goal is to recognize that their personal experiences, perceptions, and biases shape all of their decisions, including those as seemingly simple as the title of a plot. One student mentioned that she didn’t find the term “inmates” dehumanizing. I agreed with her, but I also asked whether she knew anyone who had been in jail (while making it clear she didn’t have to answer). She said no, and I admitted that I don’t either. Perhaps that’s why we don’t perceive the term as problematic. On the other hand, someone who does have a personal connection to the justice system might feel very differently.
The second goal of this exercise is to encourage students to reflect—now, throughout their studies, and later in their career—on their role as a data scientist. Is it our responsibility to use data to influence opinions, or should we simply “let the data speak for itself”? I’m not here to provide definitive answers. My goal is to encourage students to think critically as they develop into capable and responsible data scientists. To be sure, they teach me something every day and I am consistently impressed by their maturity, self-awareness, and creativity. Each fall semester, I do my best to guide them, hoping that when it’s time, they’ll spread their wings and fly on their own—confident and ready to take on the world of data science.
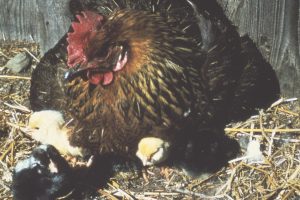
Layla Parast (center) shields and guides her fledgling freshmen—until they are ready to spread their wings and fly as data scientists…