Ryan Tibshirani wins the prestigious 2023 COPSS Presidents’ Award
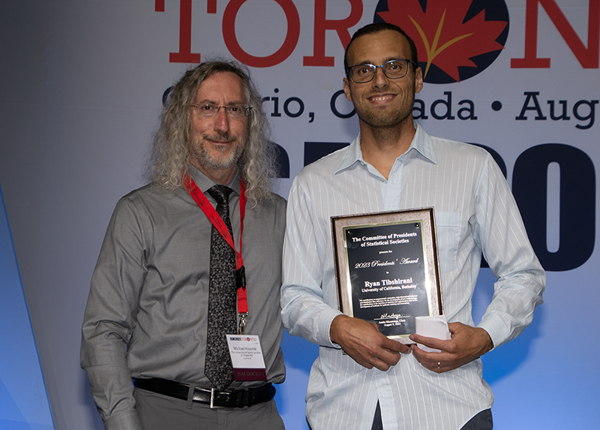
Ryan Tibshirani (right) with Michael Kosorok at the COPSS Awards session at JSM in Toronto
Ryan Tibshirani is a professor in the Department of Statistics at the University of California, Berkeley. His research interests lie broadly in statistics, machine learning (ML), and optimization and more specifically in high-dimensional statistics, nonparametric estimation, distribution-free inference, convex optimization, and numerical methods with applied interests on tracking and forecasting epidemics. Ryan completed both his BS in Mathematics and a PhD in Statistics at Stanford University.
The Presidents’ Award citation recognized Dr. Tibshirani “For contributions to nonparametric estimation, high dimensional inference and distribution-free inference; for the development of new methodology; for contributions at the interface of statistics and optimization; and for the development of methods for epidemic tracking and forecasting.”
Read on for Ryan’s interview with Amita Manatunga, COPSS Chair, and Maya Sternberg, COPSS Secretary/Treasurer.
What was your first reaction to winning the prestigious COPSS President’s Award?
Big surprise! Needless to say I am very humbled, and very grateful to my nominators.
What made you choose work in the field of statistics?
I found my way to statistics due to a mix of being inspired by my dad ([Rob Tibshirani] who is also a professor of statistics) and being drawn to the field due to my own interests and inclinations. I studied math and computer science as an undergraduate, and became interested in statistics through summer internships in biology labs, connected to my dad’s applied collaborations. There I learned the basics of data analysis “on my feet”. Initially, my interests in statistics were entirely applied. Eventually, I became aware of how broad the field of statistics is, and that being a statistician would allow me to pursue applied, methodological, computational, or theoretical questions—any of this is “fair game”. Of course, it didn’t hurt that my impressions of statisticians based on those I knew (my dad, Trevor Hastie, Jerry Friedman, and a few others) was that they are an open, curious, and fun crowd. It was then a pretty easy choice to go to graduate school in statistics.
Which part of your job do you like the most?
There is a lot to like. As much as professors might like to grumble on occasion (who doesn’t?), being a professor is a pretty amazing job. One of the most important aspects to me is intellectual freedom: having the complete freedom to pursue what you want. The way I look at the motivation behind doing research is multi-dimensional: one axis measures importance, another measures beauty. Sometimes I pursue things because I find them important, and other times because I find them beautiful or interesting. Of course, this is not to say that everything I work on succeeds in being important and/or beautiful. These are just landmarks. The point is that I get the freedom to choose my own approach.
Here are a few other things that I like about being a professor.
Advising students—this can often be a special relationship, and watching your student develop and grow can be really rewarding.
Teaching—for me, this has actually been one of the best ways to deepen my own understanding and appreciation of various topics and subfields.
Lastly, I have been incredibly fortunate with my collaborators so far. Many of my collaborators have become close personal friends, people that I would like to continue working and hanging out with for the rest of my life.
How lucky I am to have this job!
What advice would you give to young people who are entering the profession as PhD students and assistant professors at this time?
That is a tough one. There is a lot that comes to mind, but I will just share one idea. I have seen too many people in academia become distressed and unhappy, for long periods, including people that I saw at one point as persistently positive. A younger version of myself would say: “that won’t happen to me”, and would just carry on as usual, and try not to think too much about it. But my advice is now this: think carefully about your “value function”. That is, what are you using to measure the value of your work and your output (broadly interpreted), and derive a sense of fulfillment and happiness? This can be highly individual, but I believe it is worth thinking about it explicitly, and it is never too early to start (you can start as a student).
Here are two things that are meaningful to me, and that have been helpful for me to identify and keep track of: “local impact” and “mutual respect”. The first refers to the impact I have on my students, collaborators, and so on—the people I interact with regularly. I look for evidence that I am contributing positively to these “local” relationships. This makes me happy, and is more under my control than (say) where my paper gets published or whether it gets cited a lot. The second refers to the following. I start by identifying the people that I really respect. For some subset, I will be lucky enough to be able to develop a relationship with them (say, work with them). Over time, I look for signs that I may have earned their respect. Such signs can be really special.
Who are your most significant mentors? How did/do they impact your career?
At Stanford (where I was a PhD student): my dad, Trevor Hastie, Jonathan Taylor, and Emmanuel Candès. At Carnegie Mellon (where I spent the first 11 years of my faculty career): Larry Wasserman, Roni Rosenfeld, and Chris Genovese.
I can say a lot about each one and their impact. For Trevor, Jon, Emmanuel, Roni, and Chris, these thoughts will have to be saved for private conversations between us.
For my dad and Larry, I will just share a few words. My dad’s “scientific common sense” is second to none. I’ve always said to myself that if I found myself working on an applied problem of critical importance and I could bring one stats collaborator to the table with me, it would be my dad. Larry might be the closest person we have in statistics to a “universalist”. The breadth of topics that he understands (not superficially, but deeply) truly amazes me. I have learned so much from each of them, well beyond statistics. They have both accomplished so much, and yet are still so kind and generous to everyone around them. Also, they still know how to have fun, and remain young at heart.
Why were you drawn to statistical machine learning?
I think there are a few reasons. First, I am genuinely interested in computer science and optimization, outside of statistics, so it was natural for me to be drawn to machine learning. Second, ML is a fun, young field full of excitement, and this excitement can be contagious. Third, being at Carnegie Mellon had a big influence on me in this regard: though I was hired by the Department of Statistics, the people in the Machine Learning Department were welcoming from day one, and eventually I became jointly appointed in Statistics and Machine Learning. I am actually unsure of whether this would have happened anywhere else—at Carnegie Mellon, Statistics and ML are very close and collaborative. At the start of my career, I was interested in ML, but didn’t have much experience or credibility as an ML researcher. Still, the ML people welcomed me and helped nurture my interests. It was wonderful and quite formative for me.
What are your hobbies and interests beyond statistics?
Spending time with my family (I have two amazing little kids and a wonderful wife), whether it be at home or on family trips. In terms of current activities, I am mostly biking, swimming, and playing the occasional sport (I played various sports growing up and still like to dabble). I love music, and wish I had more time for it. I wish I had more time for reading books too. Maybe in the future I will make more time for music and books.
Nominations for the 2024 COPSS Awards
The Committee of Presidents of Statistical Societies will select winners for the following awards in 2024:
• Presidents’ Award
• COPSS Distinguished Achievement Award and Lectureship
• Elizabeth L. Scott Award and Lectureship
• COPSS Emerging Leader Awards
The deadline for nominations is December 15. Read more about each award, and how to nominate, at
https://community.amstat.org/copss/awards/nominations