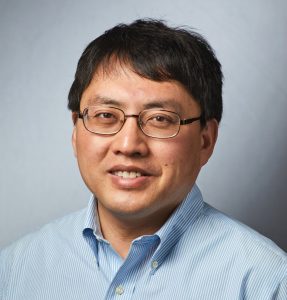
Hongyu Zhao
Hongyu Zhao received his BS in Probability and Statistics from Peking University in 1990 and PhD in Statistics from UC Berkeley in 1995. He is currently the Ira V. Hiscock Professor of Biostatistics, and Professor of Statistics and Data Science and Professor of Genetics at Yale University.
Hongyu’s research interests are the developments and applications of statistical and computational methods in molecular biology, genetics, drug developments, and precision medicine. His current projects include the analysis of biobank samples with medical records, genomics, imaging, and wearable device data, whole exome and whole genome sequencing data, single cell data, genetic risk prediction across populations, eQTL studies, multi-omics data for neurodegenerative, neurodevelopment, and psychiatric disorders, and multi-omics data for different cancers. He has published extensively with methodology papers in leading statistics, bioinformatics, computational biology, and genetics journals, and his collaborative work has appeared in leading scientific journals. Since joining Yale in 1996, Hongyu has trained over 100 doctoral and post-doctoral students. He was a Co-Editor of Statistics in Biosciences (2011–17) and Co-Editor of JASA Theory and Methods (2018–20). Hongyu has been the recipient of a number of honors, including the Mortimer Spiegelman Award for a top statistician in health statistics by the American Public Health Association, and the Pao-Lu Hsu Prize by the International Chinese Statistical Association. He is an elected Fellow of the IMS, the American Statistical Association, and the American Association for the Advancement of Sciences.
This Medallion Lecture will be given at the ENAR Spring Meeting in Nashville, March 19–22, 2023.
Statistical Issues in Genome Wide Association Studies
The past two decades have seen great advances in human genetics with the identifications of hundreds of thousands of genomic regions associated with thousands of traits and diseases through Genome-Wide Association Studies (GWAS) that collect phenotype and genotype data from large cohorts and biobanks. For example, the UK Biobank has over 500,000 participants, and the Million Veteran Program in the US has recruited close to 900,000 veterans. There are rich phenotypes (e.g. thousands of clinical traits, lab test results, imaging data, and wearable device data) and omics data (e.g. genotype data, whole exome sequencing, whole genome sequencing, gene expression, epigenetics, proteomics, and metabolomics data) available from these cohorts. These data present great opportunities for identifying functional genes and variants for different traits and diseases, inferring specific tissues and cell types relevant for a trait, characterizing the genetic architecture of complex diseases, developing disease risk prediction models that capture joint effects of genetic and environmental factors, investigating genetic similarities and differences across groups (e.g. different ancestral populations), and studying causal relationships among diseases and traits.
Despite the rich data collected from GWAS, there are many challenges in their analyses and interpretations due to the low signal noise ratios (i.e. the phenotypic effects of individual variants are relatively weak), dependence among genetic markers, complex relationships among traits, and the lack of access to individual level data for many studies. In addition, there is the need to incorporate prior knowledge on diseases and pathways, as well as the diverse sources of data generated from international efforts that can facilitate GWAS data analysis. In this presentation, we will highlight methodology developments of many collaborators and students to address these challenges in the past 10 years. We will first introduce GWAS and statistical models that are commonly used to characterize how genetic factors contribute to complex traits. We will then discuss the robustness of these models and their extensions that can help identify tissues and cell types relevant for a specific trait by integrating diverse -omics data. These models have been extended to estimate genetic correlations (both global and local) between different traits and across populations. These models have also been used for disease risk predictions using genetic and other factors. In this presentation, we will focus on the analysis of GWAS summary statistics, which are more easily accessible from GWAS, instead of individual genotype and phenotype data, a typical set up for traditional statistical analysis. The usefulness of the developed statistical methods will be illustrated through their applications to GWAS data on various diseases, including cardiovascular diseases, cancers, neurodegenerative and neurodevelopment disorders, and psychiatric disorders.