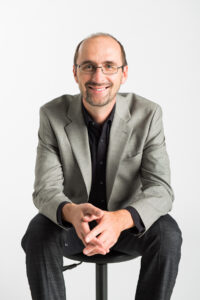
Roman Vershynin
Roman Vershynin is Professor of Mathematics at the University of California, Irvine. He works in high dimensional probability, with applications in data science. He is interested in random geometric structures that appear across mathematics and data science, in particular in random matrix theory, geometric functional analysis, convex and discrete geometry, geometric combinatorics, high dimensional statistics, information theory, learning theory, signal processing, numerical analysis, and theoretical computer science.
Roman was an invited speaker at the International Congress of Mathematicians in Hyderabad in 2010 and a winner of the Bessel Research Award from Humboldt Foundation in 2013. His book High dimensional probability: An introduction with applications in Data Science won the 2019 Prose Award for Mathematics.
This Medallion lecture will be given at the IMS Annual Meeting in London, June 27–30.
Privacy, Probability, and Synthetic Data
In a world where artificial intelligence and data science become omnipresent, data sharing is increasingly locking horns with data-privacy concerns. Among the main data privacy concepts that have emerged are anonymization and differential privacy. Today, another solution is gaining traction: synthetic data. The goal of synthetic data is to create an as-realistic-as-possible dataset, one that not only maintains the nuances of the original data, but does so without risk of exposing sensitive information. The combination of differential privacy with synthetic data has been suggested as a best-of-both-worlds solution. However, the road to privacy is paved with NP-hard problems.
This talk outlines three probabilistic approaches toward creating synthetic data that come with provable privacy and utility guarantees and doing so computationally efficiently.
This is joint work with March Boedihardjo and Thomas Strohmer.