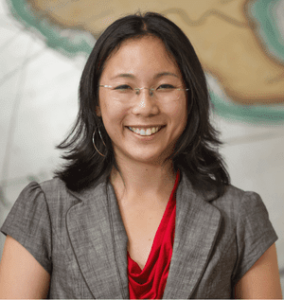
Claire McKay Bowen
Winner of the 2021 COPSS Leadership Academy and author of Protecting Your Privacy in a Data-Driven World, Claire McKay Bowen, Principal Research Associate at the Urban Institute, talks with our contributing editor Ruobin Gong about data privacy, public policy research, and data science education.
RG: Claire, thank you for taking the time to speak with me. As a statistician, what made you choose a career in public policy research?
CB: One of the big drivers for my career paths and decisions is what are the ways I can make the most impact. This is why I worked in the government initially, did internships at Los Alamos National Lab and at the Census Bureau, and went back to Los Alamos for my postdoc. To make an impact also goes into my research. From early on, I know I’m application-driven. I majored in math and physics, because math is the language of science, but I didn’t want to be a theoretician. I want to do applications in science. Through the winding path, I realized that I really like the analytical part about science. That led to statistics, where I seek for the most interesting challenges where I can make the most impact.
RG: What are some things that you enjoy most about working at the Urban Institute?
CB: Part of why I enjoy working at Urban is knowing that I’m making a difference. My work goes a step further than just the research. It translates theory into practice. Urban Institute is a non-profit, non-partisan public policy research institution. We provide evidence-based research on public policy issues, mostly in the United States and sometimes internationally. While we’re not an advocacy group, it is exciting to think that we are trying to make the society better, and life for the better.
On a day-to-day basis, my work involves a lot of writing. It is funny because, as a child, I hated writing. I went into math and science because I wouldn’t have to write! The joke is on me, as the bulk of my time now is spent on writing – not just blogs and communication pieces, but also grant proposals and full research papers.
RG: Writing is the vehicle of communication. Whatever path we choose, it seems like we can’t get away from it!
CB: Exactly. Through my undergraduate studies in physics, I got into science communication. How do I get people interested in science and understand what’s going on in science? That has also driven my current career, which is trying to communicate my research in privacy because there’s just so few of us who work in that space. If we don’t help try to communicate what’s going on in privacy, we’re not going to have the right voices at the table when important decisions are made, such as how do people get access to data amidst privacy concerns.
RG: You are a prolific writer and communicator in the privacy sphere. Your book discusses the technical challenges and also its historical, legal, and ethical aspects. In your view, how can statisticians go beyond the technical work that we do, to have our own voice, and to shape the public discourse on data privacy and other modern challenges?
CB: We should be communicating issues and ideas from data privacy to students in their early education. I talked about this in my book, that most students don’t learn about data privacy until they’re in graduate school. Even then, it’s mostly in computer science programs, except for a few statistics programs. However, a lot of discussions in the privacy community are about applications to public datasets. For instance, the new differentially private census data is at the center of much debate. What many people don’t realize is that the census data have been always altered even prior to the 2020 Census. Much of the debate suffers from miscommunication: people not knowing what’s going on. With that said, in the past, the Census Bureau did disclose data when they shouldn’t have, which contributed to a mistrust between the community and the government. This is also why the Census Bureau now takes such a strong stance on privacy. These things should have already been discussed early on in a data science education.
Another thing is, the statistics community has not been effective in advocating for our own work. The COPSS Presidents’ Award is our most prestigious award, for somebody whose career makes a significant contribution to the field. But outside of statistics, rarely have people heard of the COPSS award, whereas everybody knows what the Fields medal is. Xiao-Li Meng once said to me: “money talks”. The Fields medal was historically given with a cash prize. It was not a big amount — really small in comparison to the Nobel Prize, which awards a million dollars. But, it was still a significant amount, especially back then. People probably thought, “Oh wow, it must be prestigious.” We now have the new Rousseeuw Prize for Statistics that comes with a million dollars. I think that’ll help quite a bit to elevate statistics.
RG: What advice do you have for our aspiring data scientists who are considering a career in research and analytics in public policy and nonprofit?
CB: When people ask me what skills they should work on, I tell them it is communication. When we hire somebody at Urban, we have a minimum threshold for coding and for their statistical background. We don’t expect them to know privacy – we can teach them. But the one thing that I cannot teach as easily on the job is how to communicate with the audience, both verbally and in writing. When we interview people, we ask for a code sample and a writing sample. We ask questions such as, “Can you explain your research?” and, “How would you pitch it to a funder?”. As a nonprofit, we must be somewhat entrepreneurial in seeking funding, both through grant applications and through talking to potential funders and showcasing the kind of work that we do. So another important thing is to show passion and excitement for the work you do, which is also not easy to replicate sometimes. You want to convince other people to be excited about your work, and you should feel excited about it too.
RG: What are some exciting things that you are currently working on?
CB: I’ll tell you a technical one and a fun one. We’re working with the Internal Revenue Service (IRS) to expand access to the taxpayer dataset. Taxpayer datasets are very sensitive information, but if made accessible, the potential public policy impact is huge, because we could do more targeted decision-making, such as for stimulus packages or certain tax policy laws.
We’re constructing a synthetic dataset for public release, by balancing privacy implications with the usefulness of certain analyses. For example, if an economist wants to run a regression kink design analysis, could there be a way to apply their code on the public dataset, which would have the same structure as the confidential one?
What we’re developing is a validation server (https://www.urban.org/research/publication/privacy-preserving-validation-server-prototype, https://arxiv.org/abs/2110.12055) which has access to the confidential data. Submitting the code through the validation server returns a noisy answer. This will be an automated system. The Census Bureau had what’s called the synthetic data server through Cornell University, with two synthetic datasets and the corresponding confidential dataset. However, the queries are manually reviewed for whether the answer is noisy enough. This could take a long time if the demand exceeds staff time. Similarly, the IRS has only so many staff to maintain researcher access. An automated system would speed it up. Another motivation is that, to access many of these sensitive official datasets, you have to be a U.S. citizen. This eliminates a lot of researchers. The clearance process also takes a long time. I’ve gone through multiple of them and it doesn’t get easier.
The fun project I am working on with a colleague is called Data4Kids. It is a toolkit to help teach kids data visualization and data science. When the pandemic happened, a lot of teachers struggled to teach kids virtually. We decided to make a toolkit that could be used both virtually and in-person.
We decided to do themed data stories. We pick a dataset and modify it for different grade levels: elementary school, middle school, and high school. The data also has a messy version and a clean version. The data story is a full lifecycle. We ask: What is your data question? How do you collect the data? Is it messy? How do you analyze and visualize the data? The ending talks about data privacy, ethics and equity. We ask the students: Are you represented in this data? Would the answers from the data apply to you? Would it apply to your family and your neighbors? This way we get them to think when they hear something on the news: “Does that apply to me?”
RG: It’s wonderful to hear you talk about this. It can be difficult to get the students, especially kids, started with thinking about data equity, because the subject is so vast. But you have distilled it into a couple of simple questions that are also fundamental. “Does it apply to me?” I think this is beautiful.
CB: When people say, “Can we really teach data science to a third grader?” I think the answer is yes. We just have to frame it differently.
RG: Thank you, Claire!
Read more about Claire at https://clairemckaybowen.com/ and meet the new COPSS Leadership Academy members on page 4 of the April/May 2022 Bulletin.