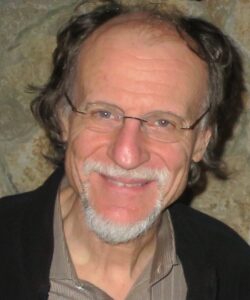
Hans-Georg Müller
Hans-Georg Müller is Professor of Statistics at the University of California, Davis. While in high school, he received the First Prize in the National Mathematics Competition of Germany (Bundeswettbewerb Mathematik) in 1974 and 1975, followed by a PhD in Mathematics from the University of Ulm in Germany and a MD from the University of Heidelberg. After deliberating whether to pursue a career in medicine or statistics, he accepted an appointment as Hochschulassistent (non-tenure track Assistant Professor) in Biostatistics at the University of Marburg in 1984 and was appointed as Associate Professor with tenure at the University of Erlangen-Nürnberg in 1987. He joined the faculty at UC Davis in 1988 as Associate Professor, was promoted to Professor in 1990 and to Distinguished Professor in 2007. His most cherished part of the job is to mentor PhD students and postdocs; 34 students have completed their PhD under his supervision. He has his best ideas while hiking in the wilderness of Northern California and scrambling in the Sierra Nevada mountain range.
He has served as chair of the Department of Statistics, founding chair of the Graduate Program in Biostatistics at UC Davis and co-editor of Statistica Sinica. Over the years he has been engaged in various biomedical research consortia, aiming to quantify brain and neurocognitive development from brain imaging data, to sequence the wheat genome, and to model aging, longevity and human mortality, with support from NIH, NSF and the Bill and Melinda Gates Foundation. His contributions to statistics include early work on smoothing methods for nonparametric regression and density estimation, methodology for growth curves and change-points, and semiparametric and structured modeling in regression. He then devoted major efforts to develop the theoretical and methodological foundations for functional data analysis, notably functional principal component analysis, empirical dynamics, time warping and functional regression, and also to build a bridge between functional and longitudinal data analysis, aligning these two areas. In his recent research he is developing concepts and methods for the emerging field of statistical analysis and inference for random objects, including distributional data analysis and transport regression. He gave an IMS Medallion Lecture in 2007, received the Senior Noether Scholar Award for Nonparametric Statistics in 2016; he is an elected member of ISI, and a fellow of IMS, ASA and the American Association for the Advancement of Science.
Hans will give this Rietz Lecture at the IMS Annual Meeting in London, June 27–30, 2022.
The Emerging Field of Random Objects and Metric Statistics
Random Objects are random variables that take values in a separable metric space, where vector operations such as addition and scalar multiplication are generally not available. Examples that are practically relevant for data analysis include samples of distributions, covariance matrices and covariance surfaces, networks and trees. With the advent of ever more advanced data recording and processing tools, such complex data are increasingly encountered, while available statistical methodology has been lacking. A special case of interest are random objects that take values in distribution spaces equipped with the (sliced) Wasserstein or the Fisher–Rao metric.
The emerging new field for the analysis of such random objects can be characterized as metric statistics. It is an area with many open and challenging problems, due to the absence of the vector space structure that underpins classical and high-dimensional statistics. Its emphasis on random objects and metric methods differentiates it from object-oriented data analysis, which was the theme of a 2010 Workshop at the Statistical and Applied Mathematical Sciences Institute (SAMSI) in North Carolina. This workshop was jointly organized by Steve Marron, Hans-Georg Müller and Jane-Ling Wang and brought the need for further research in this general area to the fore, stimulating many of the subsequent developments.
Techniques that have been developed for complex data in vector spaces, notably functional data, where the random elements are usually assumed to be square integrable and smooth random functions in a Hilbert space, are generally not applicable for random objects due to the lack of linearity. However, these techniques provide guidance of what notions to aim for (there are also some limited scenarios where functional data analysis can be more directly applied for the analysis of random objects after a linearization step). Accordingly, in the statistical analysis of random objects one aims at notions of means (barycenters, generally defined as Fréchet means), variances (Fréchet variance), conditional means and ensuing regression models (conditional Fréchet means), visualization and inference (two- and multi-sample tests).
A baseline scenario in metric statistics is the situation where the available information is limited to the distances between pairs of random objects. This scenario motivates the classical Fréchet mean and more recent extensions to conditional Fréchet means, with local, global and penalized versions of Fréchet regression providing specific implementations for the case of Euclidean predictors. Asymptotic properties can be obtained through empirical process theory. The study of Fréchet regression and more generally regression for random objects is far from complete and the presentation will include some of our recent results along these lines.
When random objects reside in geodesic metric spaces one can do more. Geodesic metric spaces of special interest include distribution spaces with Wasserstein or Fisher–Rao metrics. In these and other geodesic spaces the geodesics that connect random objects define geodesic transports that are optimal transports in the special case of the Wasserstein space. We recently introduced a transport algebra that gives rise to an intrinsic transport regression. Some other recent developments will also be discussed, including notions of depth and visualization for random objects.
This presentation is based on joint research with Satarupa Bhattacharjee, Yaqing Chen, Xiongtao Dai, Paromita Dubey, Jianing Fan, Álvaro Gajardo, Zhenhua Lin, Alexander Petersen and Changbo Zhu. Relevant preprints include arXiv:2006.13548, arXiv:2105.05439.