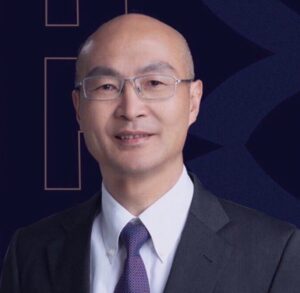
Heping Zhang
Heping Zhang is Susan Dwight Bliss Professor of Biostatistics, Professor of Child Study, and Professor of Statistics and Data Science at Yale University. He has published over 350 research articles and monographs in theory, methodology, and applications of statistics. He is particularly interested in biomedical research including epidemiology, genetics, child and women health, mental health, and substance use. He directs the Collaborative Center for Statistics in Science that coordinates major national research networks to understand the etiology of pregnancy outcomes and to evaluate treatment effectiveness for infertility.
Zhang is a fellow of the American Statistical Association and a fellow of the Institute of Mathematical Statistics. He was named the 2008 Myrto Lefkopoulou distinguished lecturer by Harvard School of Public Health and a 2011 IMS Medallion Lecturer. Dr. Zhang was the founding Editor-in-Chief of Statistics and Its Interface and is the past coordinating Editor of the Journal of the American Statistical Association.
Heping Zhang’s Neyman Lecture will be given at the IMS Annual Meeting in London, June 27–30, 2022.
Genes, Brain, and Us
Many human conditions, including cognition, are complex and depend on both genetic and environmental factors. After the completion of the Human Genome Project, genome-wide association studies have associated genetic markers such as single-nucleotide polymorphisms with many human conditions and diseases. Despite the progress, it remains difficult to identify genes and environmental factors for complex diseases — the so-called geneticist’s nightmare. Furthermore, although the impact of these discoveries on human health is not shock and awe, “drugs with support from human genetic studies for related effects succeed from phase 1 trials to final approval twice as often as those without such evidence.” Therefore, it is important and promising, while challenging, to identify genetic variants for complex human health-related conditions.
Many of us have devoted a tremendous amount of effort, or even our entire careers, to develop statistical theory and methods to meet this challenge. This talk is not intended to provide a comprehensive review of the massive progress of related methods and discoveries. Instead, I will focus on some of the work that many of my students assisted me in, over the past several years.
The first area is the identification of super-variants. A super-variant is a set of alleles in multiple loci of human genome although unlike the loci in a gene, contributing loci to a super-variant can be anywhere in the genome. The concept of super-variant follows a common practice in genetic studies by the means of collapsing a set of variants, specifically single nucleotide polymorphisms. The novelty and challenge lie in how to find, replicate, interpret, and eventually make use of the super-variants. Our work has been mainly based on the use of tree- and forest-based methods, and a data analytic flow that we proposed in 2007, which in retrospect resembles the spirit of “deep learning” that Hinton coined in 2006.
The second area is our progress in conducting statistical inference for high dimensional and structured data objects. Not only do such data objects more and more commonly appear in imaging genetic studies, but also in other areas of data science including artificial intelligence. They do not belong to a Euclidean space for which most of the statistical theory and methods such as the distribution function are developed. How do we analyze data objects in non-Euclidean spaces?
I will highlight the concepts and important properties of ball covariance and divergence, which, respectively, act as a measure of dependence between two random objects in two possibly different Banach spaces, and as a measure of difference between two probability measures in separable Banach spaces. As an example, I will demonstrate how the ball divergence was applied to study genetics of brain volumetric phenotypes.
To develop the concept of the distribution function in a metric space, we introduce a class of quasi-distribution functions, or metric distribution functions. We lay the foundation for the use of metric distribution functions by establishing the critical correspondence theorem, the Glivenko–Cantelli property, and the Donsker property for the metric distribution functions in metric spaces. The randomness of data objects can then be assessed by the distribution of metric between random object and a fixed location. Finally, the metric distribution function can play a similar role to the classic distribution function statistical inference including homogeneity test and mutual independence test for non-Euclidean random objects.
In summary, I hope to present the clear and strong motivation from the genetic and neuroimaging studies for the development of our statistical methodology and theory, share intuition and/or solid theory foundation, and demonstrate the broad and great utility of the methods to be touched on. Importantly, I hope to underscore the importance of interplay among applications, methodology, and theory in statistical research.