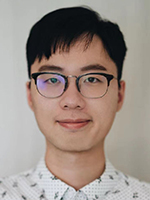
Rungang Han
Rungang Han is currently a postdoctoral associate in the Department of Statistical Science at Duke University, advised by Professor David Dunson and Professor Anru Zhang. Prior to that, he obtained his PhD in Statistics in 2021 from the University of Wisconsin-Madison, under the supervision of Professor Anru Zhang. He received a BS in Statistics in 2017 from Zhejiang University, China. He is interested in methodology and theory in high-dimensional statistics, Bayesian statistics, machine learning and non-convex optimization. His recent interest focuses on large-scale statistical matrix/tensor inference.
Rungang Han is one of the three winners of the Lawrence D. Brown PhD Student Awards, and will be presenting this lecture in a special session at the 2022 IMS Annual Meeting in London, UK, June 27–30, 2022. See https://www.imsannualmeeting-london2022.com/special-sessions for more information about the award session.
Exact Clustering in Tensor Block Model: Statistical Optimality and Computational Limit
In recent years, the analysis of tensors or high-order arrays has emerged as an active topic in statistics, applied mathematics, machine learning, and data science. Datasets in the form of tensors arise from various scientific applications, such as collaborative filtering, neuroscience, hyperspectral imaging, longitudinal data analysis, and more. Despite many recent celebrated results in statistical tensor data analysis such as tensor regression, tensor completion and tensor PCA, another important model named multi-way tensor block model has not been well studied yet. This model naturally generalizes the classic multivariate clustering models to multi-dimensional scenarios where one assumes the indexes along each dimension can be partitioned into several clusters.
While clustering analysis is prevalent in discovering heterogeneous patterns in usual multivariate data, it has unique challenges when the data is organized as a multi-dimensional array. Several extensions to high-order clustering have been developed in recent years and they typically fall into two types with different limits. On one hand, the spectral or MLE-based methods that can achieve statistical optimality are usually computationally intractable; On the other hand, polynomial-time efficient algorithms that aim at surrogate objectives (such as convex relaxation) usually suffer from the statistical sub-optimality. The tradeoff between statistical optimality and computational efficiency usually comes up in modeling low-rank tensors as it has fairly complicated algebraic structures.
In this work, we design a two-stage non-convex polynomial-time efficient procedure for the task of high-order clustering under tensor block models. At the first stage, we proposed a High-order Spectral Clustering (HSC) to initialize the clusters estimation; at the second stage, we develop an iterative scheme called High-order Lloyd (HLloyd) to further refine the clusterings. The HSC algorithm can be taken as a tensor-version spectral method and with power iterations; while the HLloyd algorithm can be seen as a high-order extension of Lloyd algorithm for 1-dimensional K-means clustering to order-d clustering. We then provide the non-asymptotic statistical properties of these two algorithms and establish the clustering error rates for the full procedure. Our proof technique features a new perturbation analysis on low-rank tensor estimation without any singular value gap assumptions.
Apart from the newly proposed algorithm and its theoretical guarantee, we discover an intriguing interplay between statistical optimality and computational efficiency of high-order clustering. Specifically, we introduce a signal-to-noise ratio (SNR) for block models which quantifies the minimum gaps between block means. This notion completely characterizes the hardness of the high-order clustering in tensor block model, which can be divided into three regions. In the strong SNR region, our method can achieve exact clustering in polynomial time; In the weak SNR region, we develop a mini-max lower bound to show that no algorithm succeeds in the task of high-order clustering; In the modest SNR region, we show that the problem is statistical possible while computational infeasible. That is, computing any estimate that achieves exact clustering is as hard as solving a version of hyper-graphic planted clique detection problem which is conjectured to be polynomial-time unsolvable. In conclusion, our theory reveals the phase transition property for multi-way clustering under tensor block model, which is further supported by the numeric experiments.
This is joint work with Yuetian Luo, Dr. Miaoyan Wang and Dr. Anru Zhang.