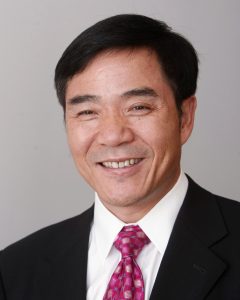
Jianqing Fan
Jianqing Fan is Frederick L. Moore Professor of Finance, Professor of Operations Research and Financial Engineering, Former Chairman of Department of Operations Research and Financial Engineering and Director of Committee of Statistical Studies at Princeton University, where he directs both statistics and financial econometrics labs. After receiving his PhD from the University of California at Berkeley, he was appointed as assistant, associate, and full professor at the University of North Carolina at Chapel Hill (1989-2003), professor at the University of California at Los Angeles (1997–2000), professor and chair at Chinese University of Hong Kong, and professor at the Princeton University (2003– ). He has served as president of the IMS and the International Chinese Statistical Association. He co-edits the Journal of Business and Economics Statistics, and was the co-editor of The Annals of Statistics, Probability Theory and Related Fields, Econometrics Journal, and Journal of Econometrics. His published work on statistics, machine learning, finance, and computational biology has been recognized by the 2000 COPSS Presidents’ Award, the 2007 Morningside Gold Medal of Applied Mathematics, Guggenheim Fellow in 2009, P.L. Hsu Prize in 2013, Royal Statistical Society Guy medal in silver in 2014, Senior Noether Scholar Award in 2018, and election to Academician of Academia Sinica and fellow of American Association for Advancement of Science, IMS, ASA, and the Society of Financial Econometrics.
Jianqing will give this Le Cam Lecture at the Joint Statistical Meetings in Seattle, August 7–12, 2021.
Read about Lucien Le Cam here.
A Theory of PCA and Spectral Clustering
Principal Component Analysis (PCA) is a fundamental tool in statistics and machine learning. Its applications range from factor analysis and tensor decomposition to blind deconvolution and manifold learning. The computational efficiency and statistical accuracy make PCA a top choice for analyzing massive data. While existing study of PCA focuses on the recovery of principal components and their associated eigenvalues, there are few precise characterizations of individual principal component scores that yield low-dimensional embedding of samples. Since all the downstream tasks count on the quality of embedding, the lack of investigation hinders the analysis of various spectral methods for community detection, clustering, ranking, synchronization and so on.
To analyze the performance of spectral methods, one often relies on the uniform () control of errors across individual principal component scores. However, uniform control over all entries often leads to vacuum bounds if the sample size is too small or the signal is too weak. In that case, one can only hope to establish bounds for a reasonably large proportion of the entries based on more refined analysis. In this talk, we first develop an perturbation theory for a hollowed version of PCA in reproducing kernel Hilbert spaces which provably improves upon the vanilla PCA in the presence of heteroscedastic noises. Through a novel analysis of eigenvectors, we investigate entrywise behaviors of principal component score vectors and show that they can be approximated by linear functionals of the Gram matrix in norm, which includes and as two special cases. The entrywise analysis is formalized via the powerful leave-one-out decoupling technique.
We apply the newly developed perturbation theory to sub-Gaussian mixture models for clustering analysis and contextual stochastic block models for community detection. Intuitively, stronger signal allows for larger p in the analysis and makes tighter error control possible. For the sub-Gaussian mixture model, our choice of p depends on the signal-to-noise ratio characterized by the separation between components, the sample size and the dimension. This adaptive choice yields optimality guarantees for spectral clustering. The misclassification rate is explicitly expressed as a simple exponential function of the signal-to-noise ratio, which implies exact recovery as a specific example. Perhaps surprisingly, the analysis reveals intimate connections between the fully unsupervised spectral estimator and Fisher’s linear discriminant analysis, which is a supervised classification procedure. Our results significantly improve upon prior arts which mostly focus on more complicated algorithms such as semidefinite programs or impose extra restrictions on the dimension and the signal strength.
In the contextual community detection problem, one observes both the network connections of nodes and their attributes. The network connections are modeled through a stochastic block model and the node attributes are modeled through a Gaussian mixture model that is independent of the network given the communities. The theory and linearization of eigenvectors lead to a tuning-free aggregated spectral estimator that is conceptually simple and computationally efficient. Remarkably, it adaptively integrates the two sources of information based on their relative signal strengths. The estimator achieves the information threshold for exact recovery and has an optimal misclassification rate below that threshold. Moreover, our results readily imply optimal spectral clustering for the stochastic block model and Gaussian mixture model separately. Simulation experiments lend further support to our theoretical findings.
This is joint work with Emmanuel Abbe (EPFL) and Kaizheng Wang (Columbia University). The manuscript is available at arXiv:2006.14062.