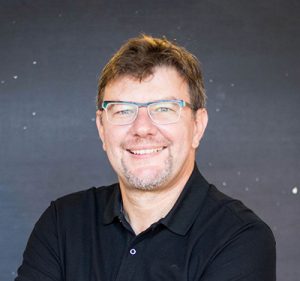
Radu Craiu
Radu V. Craiu, our newest Contributing Editor, is Professor and Chair of Statistical Sciences at the University of Toronto. He studied Mathematics at the University of Bucharest (BS 1995, MS 1996), and received a PhD from the Department of Statistics at The University of Chicago in 2001. His main research interests are in computational methods in statistics, especially, Markov chain Monte Carlo algorithms (MCMC), Bayesian inference, copula models, model selection procedures and statistical genetics. He is currently Associate Editor for the Harvard Data Science Review, Journal of Computational and Graphical Statistics, The Canadian Journal of Statistics and STAT – The ISI’s Journal for the Rapid Dissemination of Statistics Research. He received the 2016 CRM–SSC prize and is an Elected Member of the International Statistical Institute.
We live in interesting times. The initial excitement produced by the successful application of machine learning algorithms in speech and image recognition has blossomed into a veritable scientific revolution. No one remembers or cares whether the writing was on the wall, as by now it’s everywhere. The abundance of ideas and, naturally, related papers is impressive and with the power of 2020 hindsight we now realize that it has taken the machine learning community and its adjacent disciplines by surprise.
The NeurIPS conference grew in just a few years from fewer than 800 participants to more than 8000, with over 3000 registration tickets being sold in 38 seconds. Other top conferences in ML (ICML) or artificial intelligence (AISTATS) followed suit. None of these organisations were prepared to handle the immense volume that was precipitously lavished upon them. The acceptance rates for conference papers have plummeted, while social media is abuzz with communications from frustrated researchers who see their papers summarily rejected after reviews of questionable quality. Even in the Good Old Times of Yore, we all experienced the occasional lack of understanding from our peer reviews, but the new order has crystallized the notion that, simply put, there aren’t enough peers for all these reviews!
Talking to statisticians, applied mathematicians or non-ML computer scientists, one hears a lot of grumbling about transfer of resources from their disciplines and neglect of fundamental research that until yesterday supported the type of models that machine learners had built their successes on. Students migrate in droves from mathematics, statistics and other computer science sub-disciplines to machine learning, while many young researchers feel that the fastest way to glory is not through painstaking theoretical work, but rather algorithmic manipulations that lead to instances of successful predictions. It is unfortunate that the many who chose this path fail to see the intellectual leaders of the ML field who are working in a very different mode, one in which human intelligence and careful thinking, backed by principled inference, fuels the artificial intelligence. In this intense race towards a rather vaguely defined goal, the discipline most under-served—or, worse, harmed—is ML itself. One does not need to dig deep to find out that the consequences are hurting the community at all levels.
On the student side, I was recently astounded to learn from Andreas Madsen’s article in Medium, “Becoming an Independent Researcher and getting published in ICLR with spotlight,” that a young undergraduate aspiring to enter a good PhD program in ML must have already published two papers in the proceedings of some of the top conferences of the field. In other words, one must produce a significant part of a PhD thesis before entering a PhD program! The incredibly high hoops one must jump through in order to enter the academic world will likely deter many talented people from pursuing this path. In turn, this lack of formal training that reinforces a principled approach to scientific investigation threatens to dilute the impact of future methodological contributions.
On the faculty side, especially the pre-tenure kind which makes up the largest part of the ML academic community, the avalanche of papers published daily on arXiv, bioRxiv, etc., makes it nearly impossible to keep up with the literature. (A search on Google Scholar for “machine learning arxiv” yields 10,500 results, just in 2020.) “Nobody reads nor cites, just writes” is being repeated to me ad nauseam. The contents of prestigious ML conference proceedings, once reliable sources of good papers, are increasingly contested due to the lack of reviewers. In a recent Science interview, the famous statistician and prominent debunker John Ioannidis warns that the two tenets of academic publishing, credit and responsibility, are in serious jeopardy because of publication inflation. This may be merely anecdotal for tenured faculty, but it can be life-altering and health-damaging for pre-tenure researchers.
On the more practical aspects of research applications in industry, there is no doubt that the initial successes of ML were spectacular. Carefully designed algorithms have been able to win at Go, translate between languages, predict the next word I am about to type and, by and large, produce a general sense that humanity is about to win at life or, at least, reinvent life itself (see Yuval Noah Harari’s 2018 book, 21 Lessons for the 21st Century). Since those initial successes, the well-known adage, “When you have a large hammer, every problem looks like a nail” has come to describe the modus operandi for much of the industry-related data-driven research. Problems associated with moderate data volumes – usually handled with a careful statistical analysis – are now lumped together with problems that benefit from millions, or billions, of data points and are handled using similar algorithms. The potential for spectacular/costly errors is growing fast and one wonders where it will strike first (not if…).
Is there a single solution to all this? Not really. If we have to start somewhere, maybe a call to the ML community-at-large to turn their attention to foundational issues is a good start. If taken seriously, a wider effort to set research on principled legs will come accompanied by a shrinkage of propensity for publication, an increase in the standard of proof and more self-restraint in grandeur claims. The Data Science ecosystem would benefit from similar efforts in adjacent disciplines. Statisticians started this a while ago, and continue to re-examine foundational issues in various forms and forums, a good example being the BFF series of conferences (with its next stop in Toronto: see poster [below] and more info at http://www.fields.utoronto.ca/activities/19-20/BFF7). We look forward to hosting and learning from statisticians, probabilists, computer scientists and philosophers who will discuss the different statistical paradigms — Bayesian, frequentist and fiducial — for conducting sound data science. And we promise to not put out any conference proceedings!