Contributing Editor Xiao-Li Meng writes:
As might have been anticipated (jinxed?) by my thesis title, “Towards complete results for some incomplete problems,” self-pity for being incomplete has never left me. This is as true now as it was back when I accidentally reduced my almost complete thesis to merely its title, exactly 10 days before it was due. All 12 LaTeX files, one for each draft chapter, displayed zero bits on that almost fatal morning, after a 2 a.m. attempt at creating a backup copy reversed its direction. A painful lesson learned: data augmentation with sleepy or closed eyes should not be attempted. But God obviously had more lessons for me: a DVI file was left for me to build imputation. Imputation is never perfect, but I did graduate in time.
Since that time, imputation has become a source of self-help whenever my feeling of incompleteness fails to entertain itself, for reasons that are known or, otherwise, in need of rational imputation themselves. I imputed unobserved data, biased responses, latent variables, counterfactual fantasies, hidden agendas, implied ideologies, unspoken threats, suspicious motivations, and of course, the hardest of all, blinded wine labels. I imputed sometimes with deep satisfaction, and other times with deep regret. I even multiply imputed.
Never in my wildest imagination, however, had I contemplated the possibility that imputation could transform me into a time traveler — never, that is, until I encountered, a few years ago, an ingenious reporter who wrote about a comparative study on voting behaviors of politicians. The researchers couldn’t make direct comparisons among all the politicians studied because very few voted on all the legislation: politicians obviously cannot participate in voting before they were elected, or after completing their terms. The researchers therefore built a model to impute what these impossible votes would have been had these politicians been in office at the time of voting. In effect, the reporter observed, the researchers were building a time machine, allowing the politicians to travel back and forth in time to cast their votes.
Regardless how skeptical we are (as we all should be) about the validity of such an imputation model, we should admire the reporter’s creativity in coming up with a vivid analogy to arouse the public’s curiosity about something rather technical, or at least to remind the reader that there is something here both remarkable and questionable. No statistician has ever used “time travel” to describe imputing counterfactuals, despite it being a rather effective and engaging analogy. Indeed, collectively, we statisticians have done a regrettable job in coming up with rhetorically attractive means of engaging those beyond the already converted. The italic emphasis here is to remind ourselves that “regret” is even a technical term for us!
And this is not the only R-word in our vocabulary. We also have “regression”, “risk”, “rejection”, “residual error”, etc.; and speaking of error, we have another rich collection: “type 1 error”, “type 2 error”, “standard error”, “standard deviation”, “absolute deviation”, “variance”, “bias”, “mean squared error”, and the most depressing of all, “total error”… I have to wonder how many other fields would knowingly adopt a term that may leave the impression of total wrongness?
Of course, I am as guilty as anyone, for I coined “uncongeniality” as a technical term (initially for describing a thorny issue for multiple imputation, now more broadly for pre-processing).
Science and statistics are serious businesses, and as such, we should resist any temptation of creating hype terms merely for their soundbite value. At the same time, we have to admit that no matter how much we complain about deep learning without deep understanding, the phrase “deep learning” is far more likely to attract our attention than, say, “multi-layer adaptive non-linear function compositions” or MLANFC.
We should stop lamenting how other professions repackage our methods, and start doing it ourselves properly, to better engage the broader data science community and beyond. This is not an easy task, because most of us are not trained to appreciate the important roles of branding and marketing in scholarly products and dissemination, especially in an era of progressively shorter attention spans.
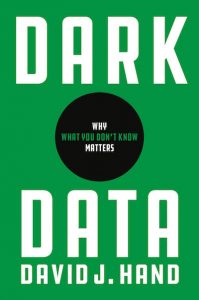
Xiao-Li Meng has been enlightened by David Hand’s “Dark Data”
I am therefore particularly excited about my fellow columnist David Hand’s (yet another) new book, Dark Data. Right away, without reading any text, you can tell that this is a book about data we cannot see but matter. Indeed, David was inspired by dark matter: “Since we can’t see this extra mass, it has been called dark matter. And it can be significant (I almost said ‘it can matter’).” The first time I saw the title, my immediate reaction was to kick myself for being so incomplete – how could I have never thought about such a catchy and apt term, especially given my years of messing with missing data, non-responses, and latent variables, all forms of dark data???
I calmed my statistical ego down (sadly) by comforting myself with the thought that, “Well, this must be another CS term.” I googled and found the term indeed has been used in the CS community, but it was used exchangeably with “dusty data.” Hats off once more to my CS friends, for “dusty data” is another clever and vivid term, which describes data that are never processed or analyzed, effectively making their collection an expensive process for gathering dust.
However, “dark” and “dusty” are not exchangeable, semantically or visually. David’s use of “dark data” is much more appropriate and comprehensive, despite his emphasis that his list of types of dark data is necessarily incomplete. David discusses 15 types of dark data, and why and in what ways they matter. He shows that they must be dealt with even if they are invisible (especially to untrained eyes). In David’s taxonomy and notation, the various forms and conditions of dark data are as follows:
DD-Type 1: Data We Know Are Missing
DD-Type 2: Data We Don’t Know Are Missing
DD-Type 3: Choosing Just Some Cases
DD-Type 4: Self-Selection
DD-Type 5: Missing What Matters
DD-Type 6: Data Which Might Have Been
DD-Type 7: Changes with Time
DD-Type 8: Definitions of Data
DD-Type 9: Summaries of Data
DD-Type 10: Measurement Error and Uncertainty
DD-Type 11: Feedback and Gaming
DD-Type 12: Information Asymmetry
DD-Type 13: Intentionally Darkened Data
DD-Type 14: Fabricated and Synthetic Data
DD-Type 15: Extrapolating Beyond Your Data
Because I initially thought that David’s notion of “dark data” only covers the kind of missing observations or incomplete data to which statisticians commonly refer, I didn’t fully appreciate some items on this list, for example, Type 11 or 12, on their own. I wouldn’t be surprised if the list generates a similar feeling for you. But this is why you need to read the book, and be convinced by David’s reasoning and his examples of cases in which unseen or unreported data play a critical and sometimes even a fatal role. You are likely to walk away with the feeling that the term dark data is indeed a very effective one to arouse both curiosity and suspicion, mixed with happiness that finally a great term was coined by a statistician—and sadness that the statistician is not you.
Oh, whereas I probably don’t want to be re-labelled as a Dark Data Scientist, I’m enlightened by David’s dark data, and believe my years of imputation practice can shed some light on the dark matter revealed in David’s book.
And I am sure you can too, unless, of course, you prefer to be a dusty (dark?) statistician…